The March 2, 2022 VelocityEHS Ergonomics Conference featured a ‘dream-team’ of our very own ergonomics, industrial hygiene and EHS technology experts alongside leading ergonomics process owners from Cummins, W.L. Gore, Lear, and Southwire, who led conference attendees through a full day of sessions covering cutting edge topics in the field of ergonomics.
During one of the afternoon sessions, Dr. Julia Penfield, Ph.D., Principal Machine Learning Scientist and Rick Barker, CPE, CSP and Principal Solutions Strategist for Ergonomics at VelocityEHS offered attendees an in-depth look at how emerging Machine Learning (ML) technologies are changing the way ergonomists assess and control musculoskeletal disorder (MSD) risks in the workplace.
Click here to watch the session on-demand!
Machine Learning Basics
The session kicked off with Dr. Penfield providing a brief introduction to ML concepts to help attendees gain a firmer understanding of this often complex data science. She covered the basics of how ML is steadily increasing our ability to make sense of vast and seemingly shapeless volumes of data. One of the key takeaways included how ML is being used to help EHS professionals answer some of their most pressing questions, including:
How do I identify the data I’m looking for?
One of the great values that ML offers is the ability to automate data classification and categorization. Basically, it automates the decision making processes around whether a data point belongs in a certain category (e.g. Is this A, or is this B?)
A common and simple example of this is Captcha verification images on certain websites, where a site may ask users to identify all images that contain a specified object, whether it’s trees, cars, stoplights, bridges, etc. The website uses this for security purposes but at the same time, the collective responses from users are training the ML model to more accurately identify those objects.
Within the scope of EHS, this categorization capability allows users to apply a ML model to documents like incident reports or safety data sheets (SDSs). This helps to identify and index specific types of information of interest. For example, we can train a ML model to find and compile all injuries of a certain type or identify all chemicals in our inventory that have a flammability hazard.
How much? How many?
Along similar lines, ML models can quantify the occurrence or frequency of events by type or any other variable that we choose. Not only that, but ML can use regression algorithms to forecast the statistical likelihood that an event may occur.
Is something is unusual or anomalous?
In other instances, we want to know if something we’ve measured is statistically anomalous compared to normal or expected levels. ML can employ anomaly detection algorithms to comb through vast volumes of measurements, instrument readings or other data points to see if there are any significant variances or outliers in the data and flag those for data users. From there, we can begin to ask why and, ultimately, how to reduce or eliminate those variances.
A great example of this is in air emissions monitoring. For many companies who have compliance obligations under EPA Clean Air Act (CAA) or similar environmental laws, their continuous monitoring systems (CMS) are collecting emissions as frequently as every hour, minute or even as often as every ten seconds. Detecting variances caused by faulty emissions control equipment, unexpected environmental releases or permit exceedances would normally require us to watch that stream of emissions data like a hawk, placing heavy time and administrative burdens on EHS personnel. ML can eliminate these burdens by analyzing data, often in real-time, to identify variance based on the statistical thresholds we plug into the model. Other potential applications include indoor air quality/workplace exposure monitoring for industrial hygiene, or identification of trends in incident rates or other key EHS indicators.
How is something organized?
Up to this point, Dr. Penfield provided examples of ML models that employ supervised learning in which users know the correct answers to the questions we’re asking the ML model to answer, and effectively guide or supervise the training of the ML to get better and better at answering correctly. With unsupervised learning, the user does not necessarily know the correct answer to the question they want to know, but we have a wealth of data available to help us answer that question. Applying unsupervised learning and clustering algorithms to the ML model can help detect patterns or clusters of data to better understand the relationships in any given scenario.
What should I do next?
This is perhaps the biggest question on the minds of EHS professionals and data users of all types. We need to be able to interpret the data we collet to help drive better decision making. As you can imagine, this is made increasingly difficult as the volume of data we’re basing those decisions on grows. ML can help by applying reinforcement learning algorithms to assess and simulate potential outcomes of a given scenario and help identify the most favorable, appropriate, or effective.
Applications of ML in EHS Management
Rick Barker, CPE, CSP, and Principal Solutions Strategist for Ergonomics led the second half of the session with a discussion of how ML can be applied in a few EHS management scenarios to help illustrate the value of ML for EHS professionals.
Ergonomics Assessment
A prime example was in the field of ergonomics management. When it comes to assessing the risk of musculoskeletal disorders (MSDs) to workers, we need to be able to collect a few important data points: the angles of joint or limb motions and postures, and the duration of those movements. The challenge previously has been inconsistency in measurement of these variables from motion to motion, and from worker to worker. This ultimately results in poor accuracy and reliability of the data that we depend on to calculate MSD risks.
To solve this problem, the VelocityEHS Industrial Ergonomics Solution introduced our Motion Capture Artificial Intelligence (AI). It uses innovative ML technology that analyzes video of workers performing job tasks, then automates the calculation of joint angles and durations throughout the performance of those tasks. It then applies additional variables to calculate the forces on different parts of the body which translates to physical strain. Not only does the motion capture system improve the speed, quality, and accuracy of the data we’re collecting, it instantly provides a composite risk assessment score and overlays that on a representation of the body to easily visualize and zero-in on MSD risks to workers.
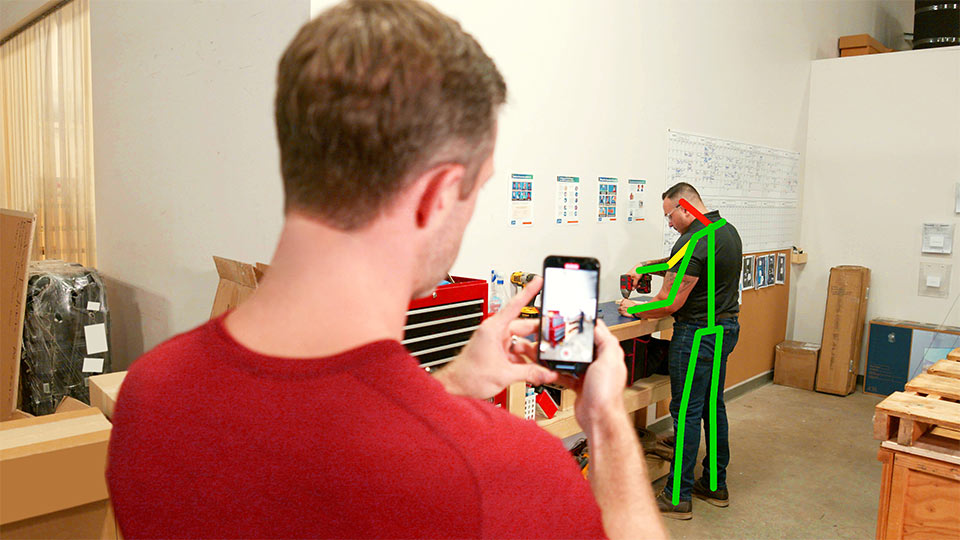
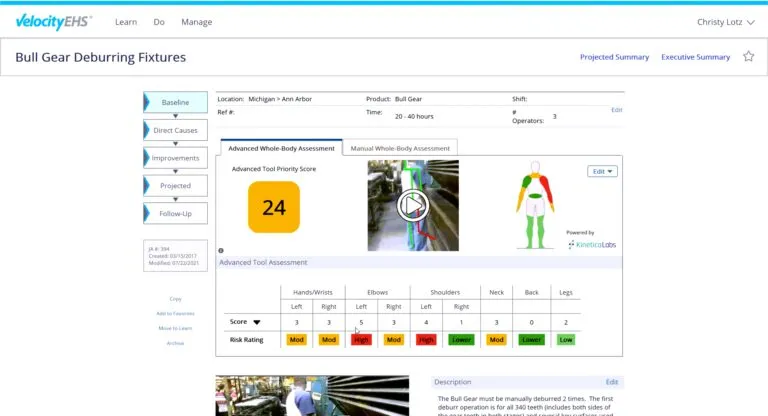
Once we’ve collected this data, and with our risk assessment findings in-hand, then comes the task of unearthing the root causes for those risks and ultimately identifying the most effective controls to manage them. The challenge here is that with potentially numerous job types involving a wide range of equipment and materials, not to mention all the other conceivable variables that figure into how jobs are performed, it can be incredibly difficult to determine true root causes for MSD risks, let alone select the most appropriate or effective controls. Unless you’re a trained ergonomist, it can be difficult to know where to start.
VelocityEHS has addressed this challenge by applying ML technology to the root cause analysis and control stages of the ergonomics management process. The system looks at the assessment data (angles, durations, job types, etc.) and based on the specific assessment findings, provides a pre-populated list that allows users to select from a list of suggested potential root causes. Custom root-causes can be entered and, over time, the system actually learns to refine the list of suggested causes based on previous selections and the root causes become more and more accurate.
The same ML technology has been applied to MSD risk controls. Based on the assessment findings and the selected root cause(s), a pre-populated list of expert-designed controls is provided. Custom controls can be added and, like the root cause analysis, ML algorithms learn over time what controls are most appropriate or effective.
Together with the motion capture assessment technology, the ML-built lists of root causes and controls provide more precise and consistent management of MSD risks. This is especially valuable for eliminating errors when assessing MSD risks and guiding non-ergonomics experts in effectively controlling those risks, while reducing the time required to manage the whole process.
Incident Management
The applications of ML to the risk assessment, root cause analysis (RCA) and control/corrective action aspects of ergonomics processes can also extend to incident management.
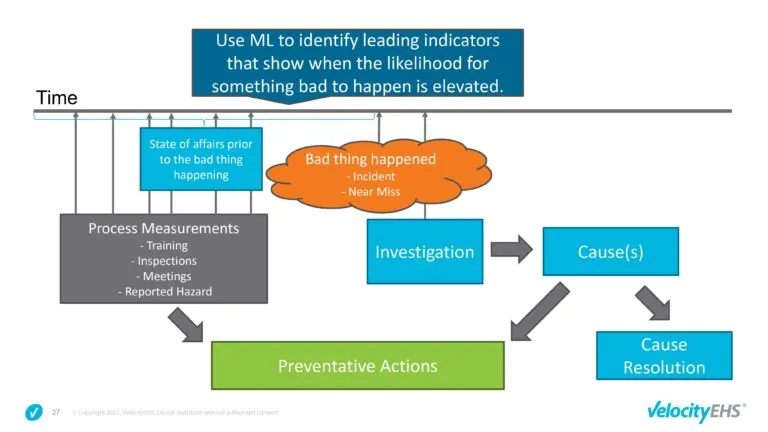
In the diagram above, we see in the middle a risk event (i.e. a workplace incident or near miss). To the left of that incident, we have information like process measurements (e.g. training activities, inspections, safety meetings, hazards, etc.) and other preventive metrics that help us to identify potential workplace risks. To the right of the risk event, we see the incident investigation, RCA and the cause resolution (e.g. controls and corrective actions).
Just as we leveraged ML to more accurately assess MSD risks during our ergonomics assessment, we can also apply ML algorithms to analyze preventive metrics prior to an incident, and identify leading indicators that can actually help EHS professionals predict the likelihood and severity of risks. Based on those risks, ML can then suggest appropriate preventive actions. If a workplace incident occurs, ML can guide EHS professionals to identify likely root causes based on our preventive metrics and other leading indicators, as well as controls that are tailored to the incident root cause(s).
Click here to watch the session on-demand!
You can also download our infographic “Emerging Technologies Changing the Industrial Hygiene Profession” as an added guide to the AI and ML technologies shaping the future of EHS.
VelocityEHS Can Help!
VelocityEHS is leading the way in ergonomics AI and ML technologies. The VelocityEHS Industrial Ergonomics solution, including our Motion Capture AI and Active Causes & Controls, is available via the VelocityEHS Accelerate® Platform, which delivers best-in-class performance in the areas of health, safety, risk, ESG and operational excellence. Backed by the largest global software community of EHS experts and thought leaders, the software drives expert processes so that every team member can produce outstanding results. For more information about VelocityEHS and its complete award-winning software solutions, visit www.EHS.com.